In recent years, artificial intelligence network behavior analysis has emerged as a crucial field in cybersecurity, network management, and data analysis. As the world becomes more interconnected and dependent on digital infrastructure, understanding how networks behave is critical to ensuring their security, efficiency, and scalability. This article explores the concept of artificial intelligence network behavior analysis, its importance, applications, and the future of this technology.
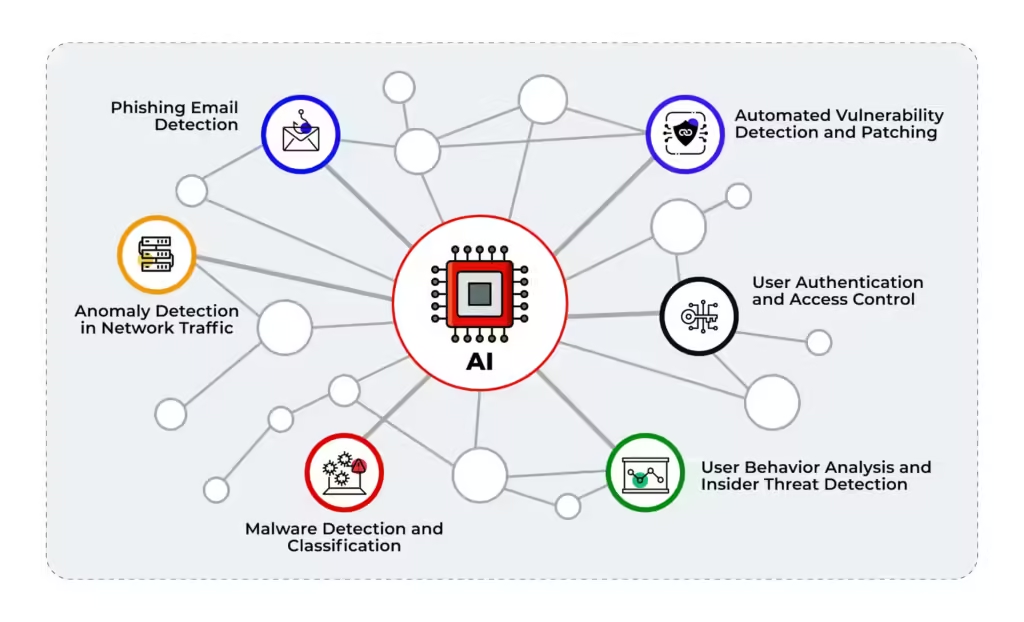
Understanding Network Behavior
Before diving into the role of artificial intelligence in network behavior analysis, it’s important to understand what network behavior entails. In simple terms, network behavior refers to the patterns and dynamics that arise when data travels through a network. These behaviors include data transmission rates, packet loss, latency, and how various devices interact within the network.
Network behavior can vary depending on many factors, such as the types of devices connected, the nature of the data being transmitted, and the network architecture. The ability to monitor and analyze this behavior is critical for network administrators, as it helps them optimize performance and identify any irregularities that may signify security threats or inefficiencies.
Why Is Network Behavior Analysis Important?
The analysis of network behavior is a critical component of modern network management and cybersecurity. It allows network administrators to detect abnormalities in data traffic that could indicate malicious activity, such as Distributed Denial of Service (DDoS) attacks, malware, or unauthorized access attempts. Furthermore, network behavior analysis helps optimize the flow of data, ensuring that networks remain fast and reliable under varying conditions.
However, traditional network behavior analysis methods often rely on predefined rules and signature-based detection systems. These methods are limited in their ability to detect new, unknown threats or adapt to evolving network conditions. This is where artificial intelligence (AI) comes into play.
The Role of Artificial Intelligence in Network Behavior Analysis
Artificial intelligence network behavior analysis leverages the power of machine learning (ML) and AI algorithms to go beyond the limitations of traditional rule-based systems. By applying AI, systems can automatically learn and adapt to network behaviors, identifying patterns that may go unnoticed by human administrators or static algorithms. This allows for more accurate and efficient detection of threats, optimization of resources, and overall better network management.
Machine Learning in Network Behavior Analysis
One of the key technologies driving artificial intelligence network behavior analysis is machine learning. ML algorithms can analyze massive amounts of network data and learn from it in real time. These algorithms don’t need predefined rules or signatures to detect anomalies; instead, they can learn what “normal” network behavior looks like and flag deviations from this norm.
This capability is particularly important in the detection of zero-day attacks—cyberattacks that exploit previously unknown vulnerabilities. Traditional security systems might miss such attacks because they don’t have a signature for them. However, an AI-driven system can detect unusual behavior that might indicate a zero-day attack, even if the specifics of the threat are unknown.
Behavioral Patterns and Anomaly Detection
AI can recognize subtle patterns in network behavior that would be too complex or nuanced for human operators to detect. For example, AI algorithms can identify patterns in how different types of traffic flow through a network, how long it takes for packets to be transmitted, and how different devices interact. These patterns can then be used to build models of “normal” behavior, which can serve as a baseline for detecting anomalies.
When the system detects activity that deviates from the norm, such as a sudden spike in traffic or unusual communication between devices, it can alert network administrators to a potential issue. This type of anomaly detection is one of the most powerful tools in artificial intelligence network behavior analysis because it enables early detection of security threats, even those that don’t have a known signature.
Applications of Artificial Intelligence in Network Behavior Analysis
The applications of artificial intelligence network behavior analysis are vast and span several industries and use cases. Below are some of the key areas where AI is making a significant impact:
1. Cybersecurity
Cybersecurity is arguably the most critical application of network behavior analysis. As cyberattacks become more sophisticated, the need for advanced, AI-driven analysis tools has become paramount. AI can help detect and prevent threats by identifying abnormal network behavior that might indicate a cyberattack in progress.
For instance, AI can recognize patterns typical of malware infections, phishing attempts, or insider threats. It can also help mitigate the damage of an ongoing attack by isolating compromised parts of the network, rerouting traffic, or alerting security personnel in real-time.
2. Network Optimization
Another significant application of AI in network behavior analysis is in the optimization of network performance. Modern networks are becoming increasingly complex, with cloud services, the Internet of Things (IoT), and mobile devices adding to the mix. Managing this complexity requires more than just human intervention; it requires intelligent systems capable of monitoring and adjusting network settings on the fly.
AI can dynamically adjust bandwidth allocation, reroute traffic, and manage congestion based on real-time data, ensuring that the network remains efficient even during peak usage periods. This type of real-time optimization is especially important for businesses that rely on high-speed internet for critical operations.
3. Predictive Maintenance
Predictive maintenance is another area where AI-driven network behavior analysis is proving invaluable. By monitoring network performance and detecting early warning signs of potential failures, AI can predict when equipment or systems are likely to fail. This allows network administrators to perform maintenance before a failure occurs, reducing downtime and preventing costly outages.
For example, an AI system could analyze network traffic patterns and determine that a certain switch is nearing the end of its lifecycle. The system could then alert administrators to replace the switch before it fails, ensuring continuous network availability.
4. Automated Network Management
AI is also enabling the automation of many network management tasks that were previously manual. These tasks include configuration management, load balancing, and even incident response. By automating these processes, AI reduces the burden on IT staff and ensures that networks are managed more efficiently.
For example, if a network experiences a sudden spike in traffic, an AI-driven system could automatically adjust settings to prevent congestion without human intervention. Similarly, if a security threat is detected, the system could take predefined actions to mitigate the threat, such as blocking specific IP addresses or isolating compromised devices.
Challenges in Implementing AI for Network Behavior Analysis
While artificial intelligence network behavior analysis offers numerous benefits, it’s not without its challenges. Some of the key challenges include:
1. Data Privacy and Security
As AI systems require access to vast amounts of data to function effectively, concerns about data privacy and security are paramount. Network behavior analysis often involves analyzing sensitive data, such as user activity logs and network traffic. Ensuring that this data is stored and processed securely is critical to avoid potential breaches.
2. Complexity of AI Models
AI and machine learning models can be highly complex, requiring specialized knowledge to develop, implement, and maintain. Not all organizations have the resources or expertise to integrate these systems into their existing network infrastructure. This complexity can create a barrier to adoption, particularly for smaller businesses.
3. False Positives and Negatives
While AI can significantly improve the accuracy of network behavior analysis, it’s not infallible. There’s always the risk of false positives, where benign activity is flagged as a threat, or false negatives, where actual threats go undetected. Striking the right balance between sensitivity and accuracy is crucial to avoid unnecessary disruptions or missed threats.
The Future of Artificial Intelligence Network Behavior Analysis
The future of artificial intelligence network behavior analysis looks promising, with advancements in machine learning algorithms, cloud computing, and edge computing paving the way for even more sophisticated systems. In the coming years, we can expect to see more widespread adoption of AI-driven network management solutions, particularly as networks continue to grow in complexity.
One emerging trend is the integration of AI with edge computing, where data is processed closer to the source rather than in a centralized location. This allows for faster decision-making and real-time responses to network events, which is particularly beneficial in industries like healthcare and autonomous vehicles, where low-latency responses are critical.
Furthermore, as AI models become more advanced, they will likely play an even greater role in predictive analytics, helping organizations not only detect issues in real time but also predict future network behaviors based on historical data.
Conclusion
In conclusion, artificial intelligence network behavior analysis represents a significant leap forward in how networks are monitored, secured, and optimized. By leveraging AI, organizations can detect threats faster, optimize network performance more efficiently, and automate many aspects of network management. Despite the challenges in implementation, the benefits of AI in network behavior analysis are undeniable, making it a critical technology for the future of network management and cybersecurity. As AI technology continues to evolve, we can expect even more sophisticated and powerful tools to emerge, ensuring that networks remain secure, efficient, and reliable in an increasingly digital world.